Why handwriting recognition was still so tricky
How Automation Hero’s AI-powered OCR handwriting solution is different — and better.
Mar 09, 2020 by Jess McCuan
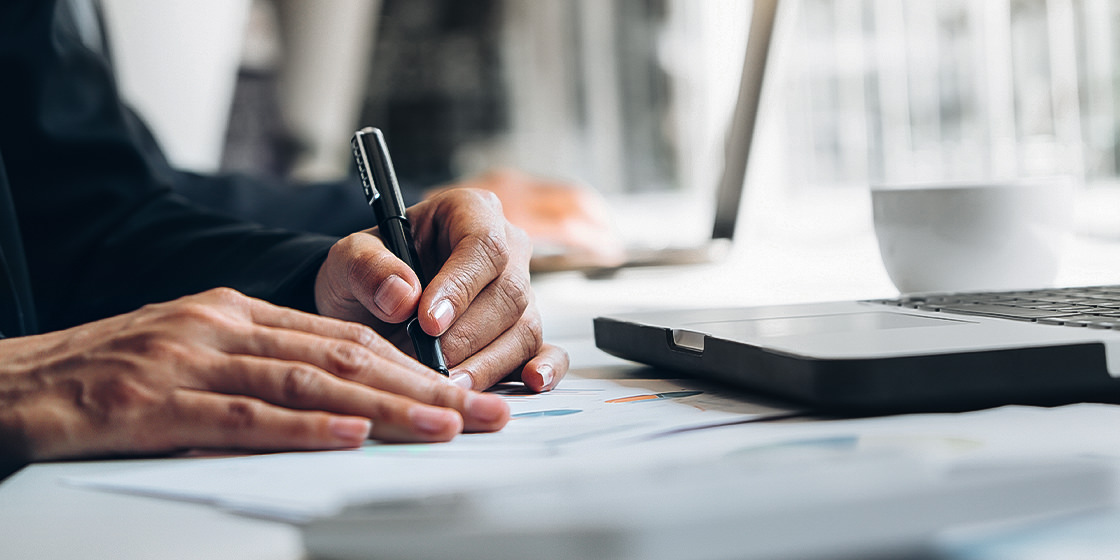
Imagine you have a rather (ahem) fluffy job: you’re a claims processor at a pet insurance company that handles policies for dogs, cats, exotic birds and other creatures. Your department can see between 400-500 claim documents per day, detailing procedures like doggie X-rays, immunizations, and all manner of exams and prescriptions.
While the patients may be cuddly, some aspects of the work get more than a little hairy. If you thought the average doctor’s handwriting was messy, try interpreting veterinarians’ notes, which cover many species and can either be fully handwritten or half computer-printed and half written, on a wide range of clinical forms. Sometimes veterinarians sign their names, while others have staff members stamp their signatures using old-fashioned paper and ink. Even to a trained eye, a phrase like “apparent lesions on paws” might be confused with “aberrant lesions on paws,” which have very different medical meanings. Pet insurers assign dozens of employees, including specialized veterinary nurses, the task of interpreting such notes. In the end, those staffers spend hundreds of hours on tedious work.
The opportunity in handwriting OCR
To help combat the problem, all sorts of companies have sprung up around handwriting OCR, optical character recognition, which converts images of handwritten text into machine-coded text to make interpretation and data entry go more smoothly. Older firms like ABBYY and IRIS started tackling OCR in the ‘80s, and now everyone from IBM to Adobe, along with smaller startups, are piling on for what will be a $13 billion market by 2025.
The trouble is, even after decades of research, most off-the-shelf handwriting recognition software lacks sophistication and can result in low accuracy rates. Especially when it comes to interpreting complex mashups of information like the pet forms above — where structured data sits side-by-side with semi-structured and unstructured data, and uncommon, colorful words get crammed into printed forms with too-small boxes.
OCR, the old-fashioned way
That’s because, while OCR software has improved, it has essentially worked on the same principles for decades. The software scans a document, weeding out artifacts and noise, like dust or stray marks. It zooms in on letters, numbers and other characters that need translating. Most software then literally “straightens up” the characters and readies them for interpretation. It evaluates each one — is this a “p”, or a “q”? — comparing it to other p’s and q’s in a stored catalog. Then it analyzes whole words. Is this “flower” or “flewer”? The program chooses “flower,” since that word exists in a stored dictionary.
Automation Hero’s OCR solution works altogether differently. The biggest difference is this: Instead of using a system that’s like a giant dictionary, looking up and comparing words against all known words in the world, Automation Hero’s OCR uses only your set of words.
Instead of using a system that’s like a giant dictionary — looking up and comparing words against all known words in the world — our OCR uses only your set of words.
Domain-specific, with AI in the lead
Our AI-powered OCR starts out using a dataset for training that’s domain-specific, i.e. unique to one particular industry or company. We can also use a practice dataset of your company’s own past documents. That means the practice dataset is highly specialized, leading to much more accurate interpretations of industry-specific words and their context. Because it saves its compute power for this narrower sphere, our OCR solution is much better equipped to accurately interpret problem cases and unusually messy handwriting.
Here’s how it works: Let’s say you’re a property insurance company. You routinely process documents dealing with the concept of “square footage.” But these words get scrawled hastily by architects and building inspectors, and your traditional OCR software is stumbling to recognize them, meaning square footage data entry is still a tedious manual process.
Our handwriting OCR solution uses a practice dataset that’s domain-specific, meaning it’s only about real estate and property. Compared to traditional handwriting recognition software, then, our OCR is more adept at recognizing variations on “square footage” and the ways architects and building inspectors might note it. It would know that “Sq footage,” “Sq feet” and “sq ftage” all mean “square footage,” for example. Or the AI might learn over time that certain letters are frequently transposed or condensed in similar ways when handwritten.
Compared to traditional OCR, our platform is more adept at recognizing variations on ‘square footage’ and the ways architects and building inspectors might note it. It would know, for example, that ‘Sq footage,’ ‘Sq feet’ and ‘sq ftage’ all mean ‘square footage.’
In any document, we also focus our attention on identifying the key area for analysis first. That means one field, box, or physical part of the page that could be trickiest. Then we apply our custom OCR approach to that specific field, again reducing the scope for false positives. In the long run, it means more information is input correctly into your company database without manual review.
Less gets lost in translation
The approach applies to material in foreign languages too. Let’s say your company regularly receives contracts in English, with a smattering of Dutch. Rather than using software that makes comparisons to all languages in the world, our OCR tool trains against a dataset that includes only English and Dutch. It would immediately be better than traditional handwriting recognition software at identifying problem cases, odd notations, or idioms in the two tongues.
If you’re already using traditional OCR software and want to keep it, no problem. Automation Hero’s platform can integrate existing OCR software, either using the translated information as a data source or zooming in on tricky cases. Because our algorithms learn fast how to handle uncommon words and edge cases, it can make quick work of documents that were previously generating errors.
Handwriting: still hanging on
For a world that’s gone digital, there’s still plenty of handwritten material around. In the United States, a country with a gaping digital divide, handwriting still rules in certain geographies. Mobile technology use is up in rural areas, but laptop and desktop usage isn’t. That means companies requiring customers to fill in longer forms — like insurers and banks — must input tall stacks of handwritten documents. Some industries, no matter where they’re located, have also simply been slow to digitize. Take construction, for example, which is awash in handwritten equipment reports. Or veterinary offices, which may be filled with messy handwritten puppy prescriptions for years to come.
Close Window
Automation Hero will track how you use the emails (e.g., at what time you open which part of the emails) sent by Automation Hero. If you have provided a separate declaration of consent that cookies for tracking your usage of the website and/or apps may be placed on your device, Automation Hero will also connect the information about your use of Automation Hero’s websites and apps (e.g., which information you open) collected by the tracking cookie to such information in so far as possible. Automation Hero will analyze such information, to identify your interests and preferences and to communicate with you in a more personalized and effective way, e.g. by providing information that you are likely interested in, like information on new technologies or products of the Automation Hero group that are likely relevant to you.